Research on Fault Prediction Model of ZPW-2000A Track Circuit Based on Improved ELM
-
摘要: 轨道电路是保证列车安全可靠运营的重要基础设备。随着铁路信号设备“故障修”向“状态修”的转变,为了对轨道电路监测信息分析进而制定科学合理的维修策略,提出一种基于 LSTM 算法的故障预测模型方法。首先,对轨道电路结构原理进行了分析,在此基础上提出使用 LSTM 模型对其故障进行预测,为验证模型的预测效果,提出用平均绝对误差(MAE)、平均绝对百分比误差(MAPE)、均方误差(NMSE)和相对误差百分比(REP)对预测结果进行度量 ;其次,结合预测实例将改进算法与传统的 SVM 算法、BP 神经网络,RNN 算法的预测效果进行对比,结果显示 LSTM 各指标均最优 ;最后,结合一个实例采用 LSTM 进行预测,验证了模型的高效性。Abstract: Track circuit is an important basic equipment to ensure the safe and reliable operation of trains. With the transition from "fault repair"to "state repair" of railway signal equipment, in order to analyze track circuit monitoring information and formulate a scientific and reasonable maintenance strategy, a fault prediction model method based on LSTM algorithm is proposed. First, the principle of the track circuit structure is analyzed, and on this basis, it is proposed to use the LSTM model to predict its failure. In order to verify the prediction effect of the model, it is proposed to use the average absolute error (MAE), average absolute percentage error (MAPE), and average absolute error (MAPE). Square error (NMSE) and relative error percentage (REP) are used to measure the prediction results; secondly, combined with prediction examples, the improved algorithm is compared with the traditional SVM algorithm, BP neural network, and RNN algorithm. The results show that the indicators of LSTM are all Optimal; Finally, combined with an example, LSTM is used for prediction, which verifi es the effi ciency of the model.
-
Key words:
- track circuit /
- LSTM /
- fault prediction /
- intelligent operation and maintenance
-
[1] 王瑞峰.铁路信号运营基础[M].北京:中国铁道出版社,2008. [2] 董昱.区间信号与列车运行控制系统[M].北京:中国铁道出版社, 2008. [3] 张友鹏,祁欢,赵斌.轨道电路分路态检测方法研究[J].铁道学报,2017,39(1):70-75. [4] 张友鹏,常高武,赵斌.基于SA算法的无绝缘轨道电路故障综合检测方法[J].铁道学报,2017,39(4):68-72. [5] 王秋实,王小敏.基于FTA与改进神经网络的轨道电路红光带诊断方法[J].铁道标准设计,2017,61(4):147-153. [6] 赵林海,刘成波,冉义奎.无绝缘轨道电路道砟电阻在线监测方法[J].铁道学报,2017,39(8):101-106. [7] 徐侃,赵林海.无绝缘轨道电路补偿电容多故障的快速诊断方法[J].铁道学报,2018(2):67-72. [8] 戴胜华,王宇琦.基于SDAE的无绝缘轨道电路调谐区故障诊断研究[J/OL].安全与环境学报:1-10[2021-04-07].https://doi.org/10.13637/j.issn.1009-6094.2020.0583. [9] 陈星,董昱.基于模糊认知图模型的轨道电路故障诊断[J].铁道科学与工程学报,2017,14(9):1983-1989. [10] 于晓英,董煜,董昱.基于多方法证据融合的轨道电路故障诊断[J].铁道学报,2021,43(2):86-94. [11] 滕金保,孔韦韦,田乔鑫,等.基于LSTM-Attention与CNN混合模型的文本分类方法[J/OL].计算机工程与应用:1-9[2021-04-07].http://kns.cnki.net/kcms/detail/11.2127.TP.20210331.1507.016.html. [12] 陈勇青.海洋环境影响下的船舶航迹预测方法研究[D].哈尔滨:哈尔滨工程大学,2019. [13] 王婷.ZPW-2000A无绝缘轨道电路故障预测研究[D].成都:西南交通大学,2015. [14] 任瑞琪,李军.基于优化核极限学习机的中期电力负荷预测[J].测控技术,2018(6):15-19. [15] 刘庆.基于LSTM模型的汽车配件安全库存量预测研究[D].成都:西南交通大学,2018. [16] 张志豪,杨文忠,袁婷婷,等.基于LSTM神经网络模型的交通事故预测[J].计算机工程与应用,2019,55(14):249-253+259. -
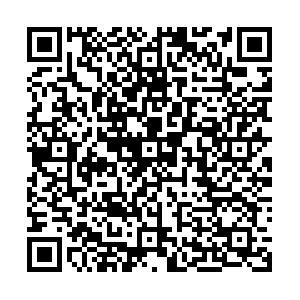
计量
- 文章访问数: 211
- HTML全文浏览量: 63
- PDF下载量: 6
- 被引次数: 0